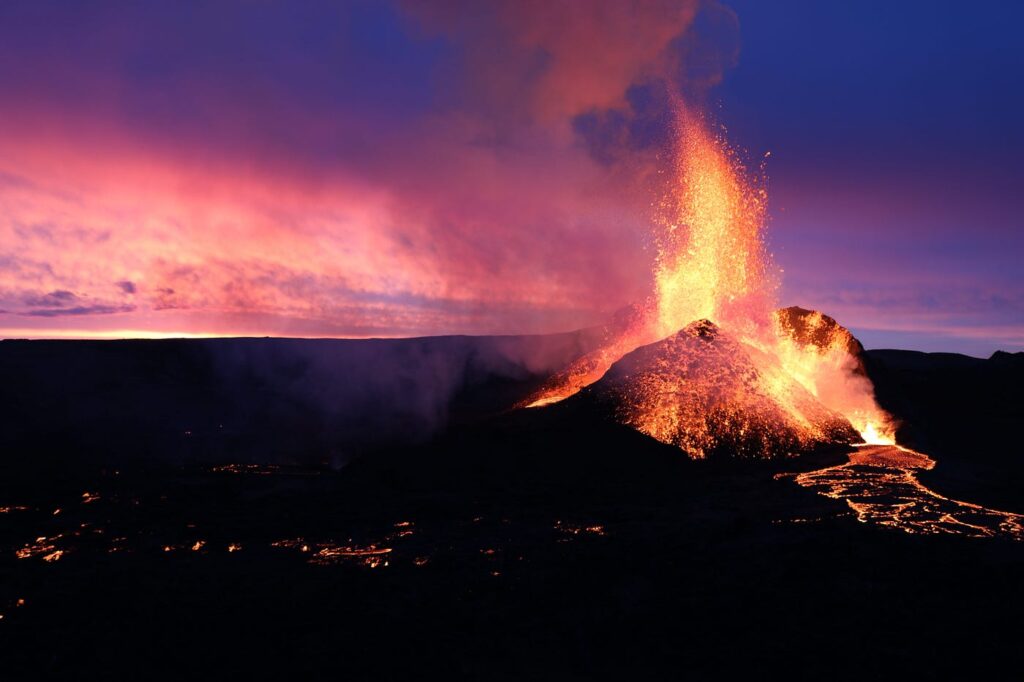
LLaVA (Large Language-and-Vision Assistant) is an advanced AI model that combines a vision encoder and large language models for general-purpose visual and language understanding. It is a novel end-to-end trained multimodal model that aims to achieve impressive chat abilities while mimicking the behavior of multimodal models like GPT-4.
The key focus of this model is visual instruction tuning, which involves using machine-generated instruction-following data to enhance the capabilities of large language models in understanding and generating content in the multimodal domain. By leveraging language-only models like GPT-4,it generates multimodal language-image instruction-following data, bridging the gap between language and vision.
With it, users can benefit from an AI-powered assistant that excels in chat capabilities and offers accurate responses to a wide range of visual instructions. It sets a new state-of-the-art accuracy on science question answering tasks and provides impressive results on unseen images and instructions.
LLaVA Architecture
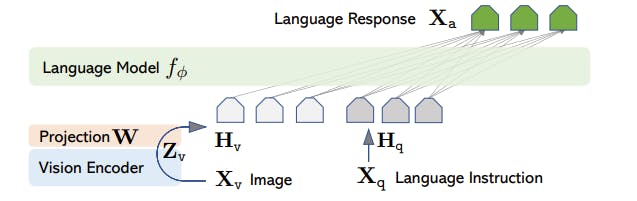
Read the original paper by Microsoft, authored by Haotian Liu, Chunyuan Li, Qingyang Wu, and Yong Jae Lee available on Arxiv: Visual Instruction Tuning.
Key Takeaways
- LLaVA Challenges GPT-4: Microsoft’s LLaVA is a powerful multimodal model rivaling GPT-4, excelling in chat capabilities and setting new standards for Science QA.
- Visual Instruction Tuning Advances AI: It’s visual instruction tuning enables AI to understand and execute complex instructions involving both text and images.
- LLaVA-1.5 Enhancements: LLaVA-1.5 introduces an MLP vision-language connector and academic task-oriented data, boosting its ability to interact with language and visual content.
- Bridging Language and Vision: It’s architecture combines LLaMA for language tasks and CLIP visual encoder ViT-L/14 for visual understanding, enhancing multimodal interactions.
References:
- encord.com
- listedai.com