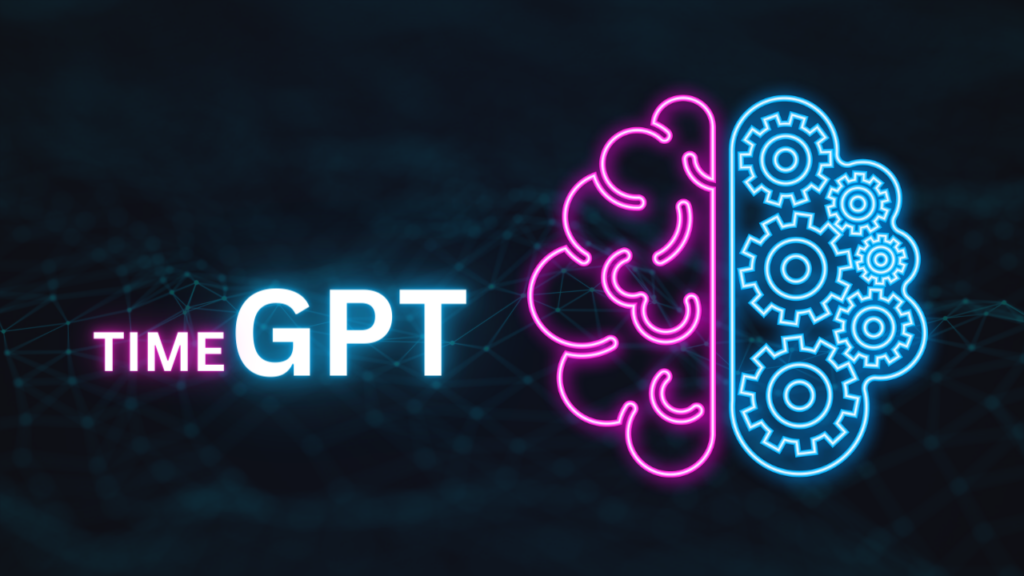
Time series forecasting is a crucial task in numerous domains, from finance and weather prediction to healthcare and marketing. Traditionally, this involved complex statistical models and machine learning algorithms that required significant expertise and computational resources. Enter TimeGPT, a game-changer in the field, introducing a powerful and accessible approach to time series forecasting.
What is TimeGPT?
TimeGPT is a generative pre-trained transformer model specifically designed for time series forecasting. It leverages the capabilities of its predecessor, GPT-3, but tailors them to the unique challenges of time series data. Instead of focusing on natural language processing, TimeGPT excels at identifying patterns and relationships within sequences of data points, enabling it to make accurate predictions about future values.
Key Features of TimeGPT:
Zero-shot inference: Unlike traditional methods that require training on each specific dataset, It can generate predictions for new data without any additional training. This makes it incredibly versatile and adaptable.
State-of-the-art performance: It has been shown to outperform established statistical, machine learning, and deep learning methods in various time series forecasting benchmarks.
Massive dataset training: Trained on over 100 billion data points from diverse sources, TimeGPT captures intricate patterns and relationships within time series data, enhancing its forecasting accuracy.
Scalability and efficiency: It can handle predictions for a single time series or millions simultaneously, making it suitable for both individual and large-scale forecasting tasks.
Ease of use: Through a simple API, It integrates seamlessly into various applications, democratizing access to powerful forecasting capabilities even for users without extensive machine learning expertise.
TimeGPT Architecture
TimeGPT’s architecture takes its cues from the Transformer model, which has proven successful in natural language processing. However, it introduces specific modifications to accommodate the unique characteristics of time series data.
First, it employs an encoder-decoder structure. The encoder meticulously analyzes historical data points, identifying crucial patterns and features. Subsequently, the decoder leverages these extracted insights to generate predictions about future values. Second, it integrates a temporal attention mechanism. This mechanism strategically directs the model’s focus to pertinent time lags within the data, enabling it to capture long-term dependencies and temporal relationships that are essential for accurate forecasting. Third, it utilizes a masked prediction approach during training. This technique involves the model predicting future values while masking them from its own input, ensuring that it learns to make inferences based solely on past data, enhancing its ability to generalize to new datasets.
Training the Time Machine
The massive dataset used to train TimeGPT is a crucial factor in its success. It covers a wide range of time series data, including financial time series, weather data, energy consumption data, and web traffic data. This diversity exposes the model to various patterns and seasonalities, enabling it to generalize effectively to new datasets.
The Future of Time-Series Forecasting
TimeGPT represents a significant leap forward in time series forecasting. Its ease of use, impressive performance, and versatility hold immense potential to revolutionize various industries. From optimizing inventory management and predicting energy demand to personalizing healthcare interventions and identifying financial market trends, TimeGPT opens doors to a future where accurate and accessible time series forecasting empowers informed decision-making across diverse domains.
Conclusion
TimeGPT is not just a powerful forecasting tool; it’s a testament to the continuous advancements in artificial intelligence. As the field of time series forecasting continues to evolve, TimeGPT stands as a groundbreaking example of how AI can unlock valuable insights from data, shaping a future where informed predictions guide our actions and decisions.