Introduction
Artificial intelligence (AI) is revolutionizing the healthcare sector, particularly in clinical development. By leveraging machine learning algorithms, natural language processing (NLP), and predictive analytics, AI can analyze vast datasets, identify patterns, and generate actionable insights faster than traditional methods. This technological synergy enables pharmaceutical companies and researchers to streamline clinical processes, reduce costs, and improve patient outcomes.
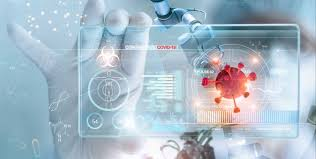
How Is AI Connected to Clinical Development?
AI has become an integral part of clinical development, offering innovative solutions to long-standing challenges. By utilizing advanced algorithms and data-driven approaches, AI enables more efficient trial designs, improved patient outcomes, and faster decision-making. The connection lies in AI’s ability to analyze complex datasets, uncover hidden insights, and support informed strategies throughout the clinical development lifecycle.
Background of AI in Clinical Development
The use of AI in clinical development has evolved significantly over the years. Initially, AI was employed to analyze clinical trial data, but advancements have expanded its applications. The integration of AI began gaining traction in the early 2000s, coinciding with the rise of big data in healthcare. Today, AI tools are indispensable in drug development pipelines, transforming how trials are designed, conducted, and monitored. This evolution underscores AI’s potential to reshape the clinical landscape.
Accelerating Drug Discovery
AI plays a pivotal role in accelerating drug discovery—a traditionally time-consuming and costly process. By analyzing molecular structures, genetic data, and biological pathways, AI identifies promising drug candidates with unparalleled speed and precision. Companies like DeepMind and Atomwise utilize AI-powered platforms to predict protein structures and screen vast libraries of compounds. These advancements not only expedite the discovery phase but also minimize the risk of failure in later stages of development.
AI in Patient Recruitment and Selection
One of the most challenging aspects of clinical trials is recruiting and selecting suitable participants. AI simplifies this process by analyzing electronic health records (EHRs), demographic data, and social determinants of health. This targeted approach ensures that trials are conducted with the right patient population, improving efficiency and diversity. Additionally, AI algorithms can predict patient dropouts and adherence patterns, allowing researchers to design more effective retention strategies.
Predictive Analytics and Risk Assessment
AI excels in predictive analytics, providing researchers with insights into potential risks and outcomes. By examining historical trial data and real-time inputs, AI models can identify adverse event probabilities, optimize dosage levels, and forecast trial success rates. This proactive risk management enhances patient safety and increases the likelihood of regulatory approval.
Generative AI in Clinical Trials
Generative AI is a game-changer in clinical trials, enabling the creation of synthetic data to augment limited datasets. This approach is particularly valuable in rare disease research, where patient data is scarce. Generative AI can simulate patient responses, predict trial outcomes, and refine study designs. These capabilities reduce the need for extensive testing and accelerate timelines, making clinical research more efficient and inclusive.
AI-Driven Data Analysis and Interpretation
The sheer volume of data generated during clinical trials can be overwhelming. AI-driven tools simplify data analysis by identifying patterns and anomalies in real time. Natural language processing (NLP) systems can process unstructured data from clinical notes, while machine learning models analyze structured datasets. This streamlined analysis aids in interpreting trial results, ensuring data-driven decision-making throughout the clinical development process.
Future Trends and Challenges
The future of AI in clinical development is promising yet complex. Emerging trends include the integration of AI with wearable devices, enabling real-time patient monitoring, and the use of blockchain for secure data sharing. However, challenges such as data privacy, algorithmic bias, and regulatory hurdles must be addressed. Ethical considerations surrounding AI-driven decisions also require careful scrutiny to ensure patient trust and equitable outcomes.
Endevsol’s AI Development Services
Endevsol specializes in developing AI solutions tailored to the unique needs of clinical development. Our expertise spans drug discovery platforms, patient recruitment algorithms, and data analytics tools. By partnering with Endevsol, organizations can harness the power of AI to drive innovation, optimize clinical processes, and achieve transformative results in healthcare.
Conclusion
AI is undeniably reshaping clinical development by accelerating drug discovery, enhancing patient recruitment, and enabling precise data analysis. As technology continues to evolve, its potential to revolutionize healthcare becomes even more evident. By addressing challenges and leveraging cutting-edge tools, the industry can unlock unprecedented advancements in clinical research. Partnering with experts like Endevsol ensures that organizations stay ahead in this AI-driven era of clinical innovation.